Chapter 32: AI, hypernudging and system-level deceptive patterns
The sudden explosion of AI tools in 2023 has received a great deal of attention from governments, regulators and tech ethicists. It is understood that AI will make misinformation and disinformation campaigns much easier. Deep fakes,1 bots,2 and a tidal wave of fake content are among the concerns.3 The impact of AI on deceptive patterns is less frequently discussed, but the themes are similar.
For example, AI could be used to assist designers in creating conventional deceptive patterns. In the same way that Midjourney4 and Dall-E5 can be used to generate images, there is a new wave of tools that can generate user interfaces from text prompts, such as Uizard Autodesigner6 and TeleportHQ AI website builder,7 as you can see in the screenshot below:8

At the time of writing, this type of tool is fairly basic, but given the rapid acceleration of AI, it’s reasonable to assume that it could become widespread soon. AI tools are reliant on training data – Midjourney is trained on millions of images from the web, and ChatGPT is trained on millions of articles. Websites and apps today tend to contain deceptive patterns, so if this new wave of UI generator AI tools are trained on them, they will reproduce variations of the same sorts of deceptive patterns unless special efforts are made to prevent them from doing so. You can imagine a junior designer giving a fairly innocuous text prompt like, ‘Cookie consent dialog that encourages opt-in’ and receiving in return a design that contains deceptive patterns. A further consequence of AI automation is that design teams will probably be smaller, so there will be fewer staff to provide critique and push back on deceptive patterns when they’re created.
In 2003, Swedish philosopher Nick Bostrom conceived a thought experiment called the ‘paperclip maximiser’. The idea is that if you give an AI autonomy and a goal to maximise something, you can end up with tragic consequences. In Bostrom’s words:9
‘Suppose we have an AI whose only goal is to make as many paper clips as possible. The AI will realize quickly that it would be much better if there were no humans because humans might decide to switch it off. Because if humans do so, there would be fewer paper clips. Also, human bodies contain a lot of atoms that could be made into paper clips. The future that the AI would be trying to gear towards would be one in which there were a lot of paper clips but no humans.’
– Nick Bostrom (2003)
Of course, this idea is currently science fiction, but if we swap the idea of a paperclip with pay per click – or indeed any kind of design optimisation based on tracked user behaviour – then it suddenly becomes a lot more realistic.
Some aspects of this idea have been around for years. For example, Facebook10 and Google11 give business owners the means to load a range of ad variations for A/B testing (measuring click-through rate or similar) and then to have the tool carry out automatic selection of the winner. This takes the human out of the loop, so the advertiser can press the ‘run’ button and then leave it to do the job. If they happen to check in after a few weeks, they’ll find that ‘survival of the fittest’ has occurred. The ads that people didn’t click have been killed off, and the ad that was most persuasive at getting clicks has become the winner, shown to all users.
These systems are not autonomous, thankfully: a human has to set it up, provide the design variations and it’s limited to just advertisements. With the new generation of AI tools, we can imagine this working at a grander scale, so a human would be able to give a broad, open-ended brief, press ‘go’ and leave it running forever – writing its own copy, designing and publishing its own pages, crafting its own algorithms, running endless variations and making optimisation improvements based on what it has learned. If the AI tool has no ethical guide rails or concepts of legal compliance, deceptive patterns are inevitable – after all, they’re common, easy to build, and generally deliver more clicks than their honest counterparts.
Related to this is the idea of persuasion profiling12 or hypernudging13 – a system that tacitly collects behavioural data about what persuasive techniques work on an individual or market segment, and then uses that knowledge to show deceptive patterns that are personally tailored to them. For example, if a system has worked out that you’re more susceptible to time pressure than other cognitive biases, it will show you more deceptive patterns that take advantage of time pressure. Research so far has focused on cognitive biases, but it’s easy to imagine it being extended to target other types of vulnerability; if the system works out you have dyslexia, it could target your weakness by employing more trick wording in getting you to complete a valuable action like signing a contract. If you have dyscalculia (difficulty with numbers and mental arithmetic) then it could target your weakness by using mathematically tricky combinations of offers, bundles and durations.
In his book Persuasion Profiling: How the Internet Knows What Makes You Tick12, Dr Maurits Kaptein argues that ‘Persuasion profiles are the next step in increasing the impact of online marketing’ and are ‘especially useful if you are trying to sell things online’. The figure below shows a persuasion profile from one of Kaptein’s research papers. Each row depicts a ‘persuasion principle’ derived from Cialdini’s ‘weapons of influence’.14 The x axis depicts whether the item will have a positive or negative effect in influencing the user’s choice.15
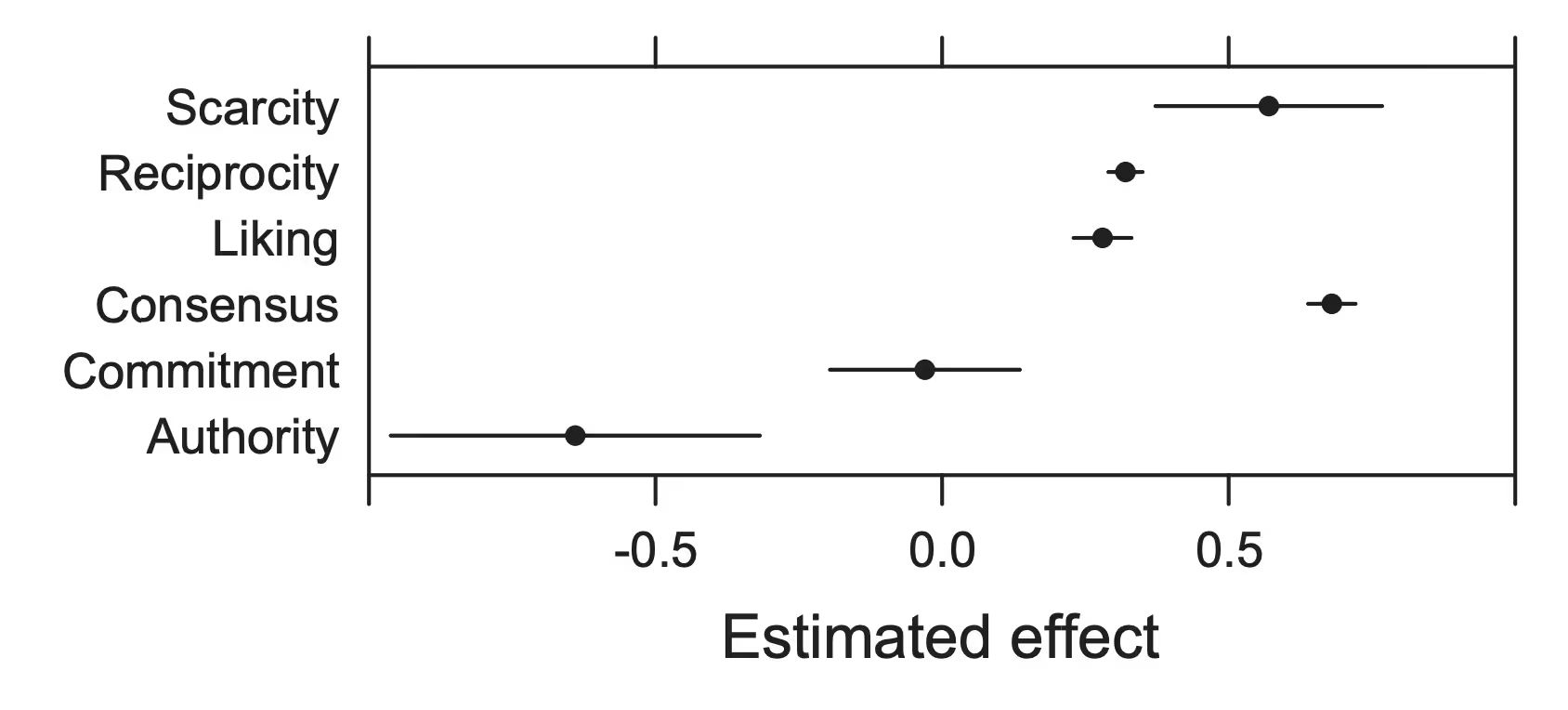
In one research study, Kaptein et al. had hundreds of participants complete a ‘susceptibility to persuasion scale’ (STPS), deriving persuasion profiles for each individual. They then had the participants carry out a dieting activity in which they were encouraged to eat fewer snacks between meals. They were prompted to log their snacking behaviour via SMS. Unbeknown to them, the prompt SMS messages contained persuasive content that was varied by experimental condition. Those participants who received messages based on their persuasion profile were persuaded more effectively than any other group – they snacked less between meals.
The concept of persuasion profiling is related to so-called psychological warfare tools and the Cambridge Analytica Brexit scandal, in which personalised political messages were covertly sent to individuals in the UK based on illegally acquired personal profiles, thereby influencing the result of the Brexit referendum.16 It seems this scandal may still be fresh in the minds of EU legislators given the enormous focus on privacy and consumer protection in legislation in recent years.
In a 2023 research paper subtitled ‘Manipulation beneath the Interface’, legal scholars Mark Leiser and Cristiana Santos put forward the concept of a spectrum of visibility of deceptive patterns.17 In other words, deceptive patterns range from those that are easily detected by an investigator, to those that are hard to detect without extensive work. Leiser and Santos summarise this spectrum of visibility into three tiers – ‘visible’, ‘darker’ and ‘darkest’, which can be unpacked and described as follows:
- Self-evident deceptive patterns: Things like confirmshaming, forced action and nagging are visible for anyone to see. They’re brash and rather unmissable.
- Hidden but UI-based deceptive patterns: Things like sneaking and misdirection are designed to be insidious and to slip by without the user noticing, but they can be detected by an investigator doing a careful analysis of the pages (that is, they are observable on the pages, which can be screenshotted and highlighted).
- Multistep business logic deceptive patterns: This type is algorithmic, but using relatively trivial logic. Imagine a multistep questionnaire where the user answers some questions, and branching logic drives them to one offer or another based on their responses. This sort of deceptive pattern can be investigated by running through the steps multiple times, and representing the behaviour as a flow chart.
- Complex algorithm-based deceptive patterns: Traditional personalisation and recommendation systems fall into this category. These algorithms involve complex code and mathematics, and the exact system behaviour cannot accurately be discerned without viewing the system source code (which is typically not available to the public). However, the behaviour is deterministic – given the same inputs, the same result is always given. This means a business can always account for the precise behaviour of their system, though the explanation would most likely involve mathematical formulae or pseudocode.
- AI-based deceptive patterns: Systems that learn from user input and employ AI (such as large language models like GPT) can be inscrutable closed boxes, even to the people who create them. The behaviour of the system is emergent and probability-based. This means that given the same inputs, the system can give different answers to different users or at different points in time. This poses a regulatory challenge because businesses will struggle to account for exactly how their system behaves towards users.
To date, most work on deceptive patterns has been focused on the uppermost visible layers, and there is much more work to be done to understand the deeper, less visible deceptive patterns.